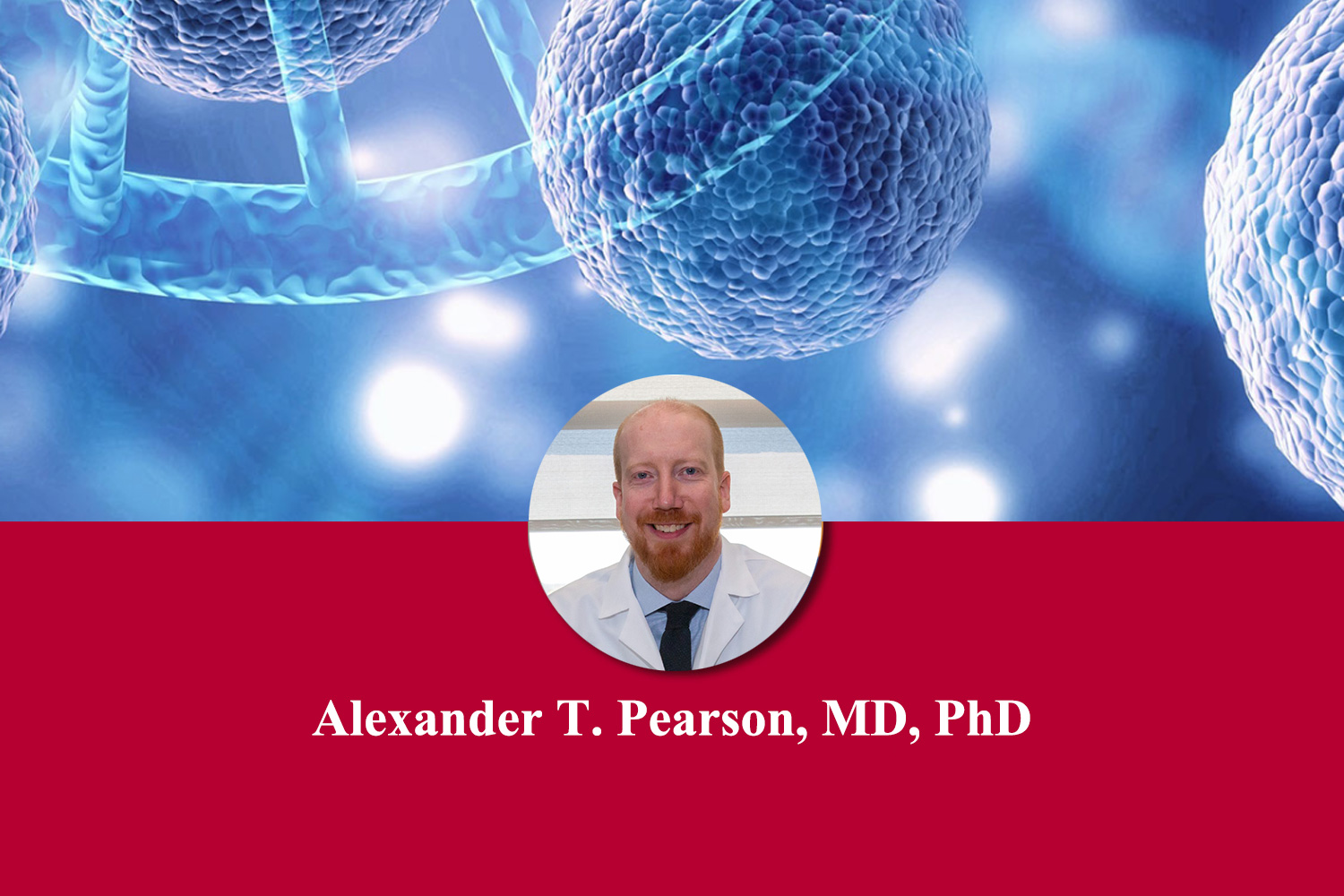
10 Dec Advancing Treatment with AI
Written by: Alexander T. Pearson, MD, PhD
The application of artificial intelligence (AI) in the treatment of head and neck cancer (HNC) has the potential to significantly improve treatment outcomes for patients. By taking advantage of the capabilities of AI to assist with diagnosis, interpretation of a patient’s imaging or cancer tissue, or predicting how a patient will respond to therapy, we will be able to adjust our management of a given patient and personalize their treatment plan to ensure they have the best chance of success.
Machine learning, which is a field of AI, is of great interest in healthcare. Machine learning focuses on using data and algorithms to enable AI to learn the way that humans do, eventually allowing a model to make decisions and predictions. To explain machine learning, consider this example. Can you tell which of these two animals below is a leopard?
To choose the leopard, you could iteratively compare the picture of a leopard and the first two images to see which one it looks most like. What might also help is if you were shown multiple pictures of leopards, allowing you to identify patterns between them. After looking through enough examples, you might notice that the cheetah on the left has round spots, whereas leopards have a distinctive “rosette” pattern. There are also fewer spots on a cheetah’s face and the eyes look a bit different. Over time, if you continued to examine pictures of both cheetahs and leopards, you would become much more confident in telling them apart.
In a way, machine learning is similar. Using computers and special software, we can provide a model with large amounts of data and information and train it to learn a particular topic. Over time, that model will be able to recognize differences and make associations, including very subtle ones that even experts might not notice. Eventually, it will be able to make decisions all on its own. This has application in our world today: businesses give data to AI models to anticipate consumer habits, helping them stay one step ahead. Social media analyzes your history and uses AI to predict what your interests are and adjusts your newsfeed based on this. However, AI is not always accurate and predictions can be incorrect.
In the HNC field, one of the goals of AI is to be able to train a model with patient data, including imaging, histological data (features of a patient’s cancer tissue), clinical data, and other available records. The model would learn to identify traits, or combinations of traits, that differentiate patients who respond well to treatment versus those who respond poorly. Why do two patients with the same disease respond differently to the same treatment? It is almost as if the model is trying to identify the rosette pattern amongst all this data and classify patients accordingly. If trustworthy, this information would change how we manage patients. We could offer a more optimal treatment plan and increase treatment for those who may respond poorly or be less aggressive with patients who have characteristics of a good responder, which could decrease long-term side effects.
The treatment of HNC is complex and relies on many different factors. A patient’s age, genetic changes in the cancer, smoking history, or where the cancer originated from can all play a role in prognosis. Other factors such as a patient’s genetic profile, whether lymph nodes are involved, or whether the cancer is related to the human papillomavirus (HPV) all provide information that impacts treatment decisions.
In addition to this wide array of patient information, the team also weighs all of the different treatment options. We discuss whether a patient should receive surgery or if they are better suited for radiation, each of which has significant tradeoffs. We carefully assess which chemotherapy drug will benefit the patient the most, as well as the dose and duration of treatment. For patients whose cancer has spread to other organs or was previously treated and comes back, there are multiple immunotherapy agents, targeted therapies, and novel clinical trials to consider. The team carefully evaluates all of these factors, and still, patients may not respond as predicted. We hope to use AI to help us analyze all of these factors and assist us in the decision-making process, which could improve results for patients.
Exciting new research
Extracapsular extension (ECE), which is when cancer cells break through the protective boundary of a lymph node and invade surrounding tissue, indicates a poor prognosis for HNC. The presence of ECE increases cancer stage and requires aggressive treatment, such as a more extensive surgery or higher doses of chemotherapy and radiation. However, ECE can only be identified by a pathologist after the lymph nodes have been surgically removed and cannot be determined beforehand on imaging. Some patients will not know that they have ECE if they never have surgery. The lab of Dr. Benjamin Kann at Dana-Farber Cancer Center is researching whether a model can be trained to analyze a patient’s CT scans to determine if ECE is present. They trained a model on pre-treatment imaging for 110 patients with or without ECE. Their algorithm and model was able to predict ECE on imaging with high accuracy.1 While the model may be refined further, the significance of this is clear. If we can predict whether a patient has ECE without having to undergo surgery, then we will be able to adjust our management of a patient sooner, which could include avoiding a risky cancer resection if other options are more favorable.
The lab of Dr. Anant Madabhushi at Emory University is exploring ways for AI to predict treatment outcomes by evaluating tumor histology slides of patients. In recent publications, the lab presented a new metric called the multinucleation index (MuNI). A model was trained to review histological images and determine key characteristics of the tumor, such as the amount of epithelial nuclei. They then calculated the MuNI score for each patient to see if it could predict patient outcomes, such as disease-free survival and distant metastasis-free survival. The MuNI was shown to have more accurate predictive ability than classically used variables such as smoking status, cancer stage, or lymph node involvement.2 The ability for AI to rapidly, and accurately, predict a patient’s outcome with a review of a single slide would be game-changing. This could alter a patient’s cancer treatment and would October 2024 Page 5 impact how closely we monitor them after treatment. A recent clinical trial showed a benefit of using immunotherapy in disease-free patients who are at high risk of recurrence.3 If the MuNI could identify patients with low disease-free survival, perhaps we could start immunotherapy soon after completion of treatment.
HPV is another prognostic factor of how a patient may respond to treatment. Studies have shown that HPV- related oropharyngeal HNC has a superior response to therapy and better survival outcomes compared to HPV-negative cancers. Additionally, HPV-driven HNC can be treated with lower intensity of chemotherapy and radiation, and can be more susceptible to immunotherapy. Determining HPV status can be done in a variety of ways. Some tests are highly sensitive, but are more expensive and time consuming. Some tests are faster, but are neither perfectly sensitive nor specific. One of the goals of our lab is to use machine learning to determine the presence of HPV in cancer tissue. In a study, we trained a model with tumor histology slides for over 400 patients with or without HPV. Our model was able to predict which slides had HPV 80% of the time.4 If our model can be improved, this would help clinicians quickly confirm HPV status rather than wait for results, which can take a couple of weeks, allowing us to confirm a treatment plan sooner and could also avoid costly testing.
The future of AI in head and neck cancer care is bright and we are seeing the first exciting implementations into practice. However, while there has been incredible progress in the last several years, we do not rely on AI comprehensively for cancer care. To achieve the best implementation of AI for HNC patient care, we will need considerable quality control to ensure models are accurate, precise, and reliable, which takes time. Further, will AI be able to consider a patient’s support system, resources, and psychosocial needs, all of which can impact the treatment plan that we recommend? This is why even with the integration of AI, clinicians will still need to use their experience, expertise, and compassion when evaluating patients, but the potential for AI to improve patient outcomes and help the care team navigate challenging situations makes this a resource worth investing in.
Editor Note: Dr. Alexander Pearson is an Associate Professor of Medicine in the section of Hematology and Oncology at the University of Chicago. Dr. Pearson serves as Director of the University of Chicago Center for Computational Medicine and Clinical Artificial Intelligence as well as the Director of the Head/Neck Cancer Program in Medical Oncology. He is a Chan-Zuckerberg Chicago Biohub Investigator and has a Joint Appointment at Argonne National Laboratory. Dr. Pearson trained as a PhD statistician and physician at the University of Rochester where he won the national American Statistical Association Student Paper Award. He then completed both subspecialty training in Hematology/Oncology and postdoctoral research in mathematical systems biology and head/neck cancer at the University of Michigan, where he was also Chief Fellow. Dr. Pearson currently practices medical oncology at the University of Chicago Comprehensive Cancer Center where he is an active clinical investigator and clinical trialist and runs multiple investigator-initiated interventional studies.
Dr. Pearson is nationally recognized in voice guiding applications of quantitative methods to cancer research, and his research lab studies clinical applications for machine learning, mathematical modeling, AI, quantum computing, and systems biology. He serves on AI-in-medicine advisory groups to the National Institutes of Health (NIH), American Society of Clinical Oncology (ASCO), and American Medical Association (AMA).